Supply chains are becoming increasingly complex, seamlessly weaving together a vast network of organizations. This intricate tapestry of interdependencies, while driving efficiency and global reach, also presents a growing concern: cyber risk. As supply chains become increasingly reliant on digital technologies, they become susceptible to cyberattacks that can cripple business operations, expose sensitive data, and inflict substantial financial losses.
The escalating threat of supply chain cyberattacks is evident in the alarming statistics:
- 97% of organizations have experienced a cyberattack that originated within the organization’s supply chain.
- In 2022, supply chain attacks affected over 11 million individuals and compromised the data of 1,743 entities.
- Another report states that the average breach lifecycle takes 287 days, with organizations taking 212 days to detect a breach initially and 75 days to contain it.
- In 2022, there were 115 claimed supply chain attacks in the United States, which is the highest reported number since 2017.
- Overall, supply chain attacks saw a year-over-year increase of 37 percent between 2021 and 2022.
- In 2022, around 11 million customers were affected by supply chain cyber-attacks worldwide.
Additionally, several commercial companies provide cyber ratings based on information about the organization accessible by outside parties, often called outside-in ratings. While these cyber risk assessment ratings can provide some insights into an organization’s cyber posture, they may not be as comprehensive or accurate as assessments that take into account the organization’s internal security practices and the security posture of its supply chain partners. Traditional methods have played a role in supply chain risk management, but they face several limitations.
To address these limitations and threats, innovative supply chain cyber risk analytics are emerging as a critical tool for organizations of all sizes. These cutting-edge solutions go beyond traditional cybersecurity measures, leveraging data-driven insights and advanced algorithms to identify, assess, and mitigate cyber risks across the entire supply chain ecosystem.
What is Supply Chain Cyber Risk Analytics?
Technically, Supply Chain Cyber Risk Analytics, also known as Cyber Supply Chain Risk Management (C-SCRM), is a process that identifies, assesses, and mitigates the risks associated with the distributed and interconnected nature of Information and Communications Technology (ICT) and Operational Technology (OT) product and service supply chains.
In simple terms, it involves gathering information about the suppliers, vendors, and partners that contribute to an organization’s products and services, as well as the technologies and processes used to manage and control the supply chain.
Supply chain cyber risk analytics helps organizations to:
- Identify and prioritize cyber risks: Organizations can identify the most critical cyber risks to their supply chain by analyzing data from various sources. This helps them to focus their resources on the areas where they are most vulnerable.
- Assess the likelihood and impact of cyberattacks: Supply chain cyber risk analytics can help organizations assess the likelihood and potential impact of a cyberattack on their supply chain. This information can be used to develop mitigation strategies and contingency plans.
- Monitor and track cyber risks: Organizations can use supply chain cyber risk analytics to monitor and track cyber risks over time. This helps them identify trends and emerging threats and take corrective action as needed.
- Measure the effectiveness of cyber risk mitigation strategies: Supply chain cyber risk analytics can be used to measure the effectiveness of cyber risk mitigation strategies. This helps organizations to identify areas where they can improve their defenses.
The Need for Innovation in Supply Chain Cyber Risk Management
As cyber threats become more sophisticated, dynamic, and diverse, traditional methods of cyber risk analytics are not sufficient to cope with them. Conventional methods of cyber risk analytics are based on the use of signatures or indicators of compromise to identify threats and rely on predefined rules or patterns that match known cyber-attacks or vulnerabilities.
However, these methods have several limitations, such as:
- They are not effective for detecting and blocking unknown or emerging threats, as they do not have signatures or indicators for them.
- They are reactive rather than proactive, as they rely on historical data and do not anticipate future threats or scenarios.
- They are prone to false positives and false negatives, as they can miss subtle variations or anomalies in the data or systems.
- They are costly and resource-intensive, as they require constant updates and maintenance of signatures or indicators.
Moreover, these methods are not well suited for the challenges posed by the increasing complexity and digitization of supply chains, such as:
- The integration of multiple systems, processes, and partners across the supply chain increases the interdependencies and vulnerabilities of the network.
- The adoption of emerging technologies enables new capabilities and opportunities but also introduces new risks and uncertainties.
- The demand for higher levels of service, quality, and customization from customers requires more agility, flexibility, and responsiveness from the supply chain.
Therefore, there is a need for innovation in cyber risk analytics, using advanced methods and technologies that can overcome the limitations of traditional methods and enable organizations to enhance their supply chain security and resilience.
Innovative Solutions for Supply Chain Cyber Risk Analytics
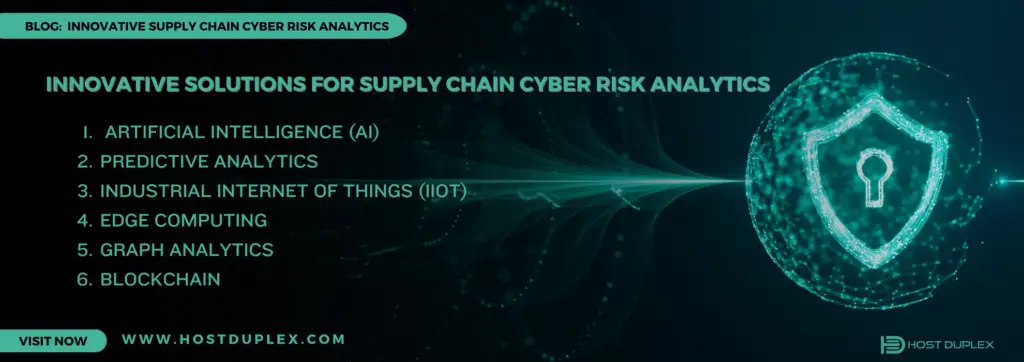
1. Artificial Intelligence (AI)
AI has been increasingly applied to cyber risk analytics, as it can help organizations improve their cybersecurity posture, detect and prevent cyberattacks, and respond to incidents more efficiently and effectively. AI can help supply chain organizations to address cyber risks in several ways, such as:
- Cyber risk identification and assessment: AI can analyze large amounts of data from various sources, such as suppliers, customers, sensors, devices, systems, etc., to identify potential cyber threats and vulnerabilities within the supply chain. AI can also assess the likelihood and impact of cyber risks and prioritize them based on their severity and urgency.
- Cyber risk mitigation and prevention: AI can provide recommendations and solutions for mitigating and preventing cyber risks, such as patching, updating, encrypting, isolating, backing up, etc. AI can also automate some of these actions or assist human operators in executing them. AI can also monitor the effectiveness of the mitigation and prevention measures and adjust them as needed.
Some examples of AI applications for supply chain cyber risk analytics are:
- Scenario modeling: AI can help supply chain organizations model and simulate various cyber risk scenarios and evaluate their potential impacts and outcomes. This can help to test and validate the cyber risk management strategies and plans and identify the best practices and solutions. For example, Accenture has developed an advanced scenario modeling tool that uses AI to generate realistic and relevant cyber risk scenarios for supply chain organizations and assess their impacts on key performance indicators, such as revenue, cost, customer satisfaction, etc.
- Unified demand planning: AI can help supply chain organizations integrate and harmonize the demand signals from different sources, such as sales, marketing, operations, finance, etc., and create a unified and accurate demand forecast. This can help to optimize the supply chain planning and execution and reduce uncertainty and variability. AI can also help to detect and prevent any cyber risks that may affect the demand planning process, such as data manipulation, sabotage, fraud, etc.
- Supplier risk monitoring and resolution: AI can help supply chain organizations monitor and evaluate the cyber risk posture of their suppliers and identify any issues or anomalies that may indicate a cyber risk. AI can also help resolve supplier cyber risks by providing alerts, guidance, and support for supply chain organizations and their suppliers. AI can also help to improve the collaboration and communication between the supply chain organizations and their suppliers and enhance trust and transparency.
2. Predictive Analytics
Predictive analytics is emerging as a cornerstone in the foundation of supply chain cyber risk management. By harnessing vast amounts of data and employing advanced statistical models, machine learning, and AI algorithms, predictive analytics provides foresight into potential cyber threats and vulnerabilities within supply chains.
The process begins with the aggregation of extensive data sets, including historical cyber incident records, current threat intelligence, and network traffic patterns. Predictive analytics tools then analyze this data to identify patterns and correlations that might otherwise go unnoticed. These patterns help in predicting the likelihood of future cyber threats and their potential impacts.
3. Industrial Internet of Things (IIoT)
The Industrial Internet of Things (IIoT) has revolutionized supply chain operations, introducing a new level of connectivity and data exchange that has significantly improved efficiency and productivity. It enables real-time monitoring and control of physical systems and facilitates interaction between humans and Cyber-Physical Systems (CPS). This integration of IIoT theories and control of physical systems can help businesses embrace the opportunities from Industry 4.0 (I4.0), enhancing and automating their business processes.
With the integration of IIoT in supply chains, there is an increased focus on mitigating cyber risks. A dynamic and self-adapting supply chain system supported by Artificial Intelligence and Machine Learning (AI/ML) and real-time intelligence for predictive cyber risk analytics has been identified. This system is integrated into a cognition engine that enables predictive cyber risk analytics with real-time intelligence from IoT networks at the edge.
4. Edge Computing
Edge computing is one of the innovative methods in cyber risk analytics that can improve the security and performance of supply chains. Edge computing refers to the processing and analysis of data at the edge of the network, near the source of data generation, such as IIoT devices, sensors, and machines.
The integration of edge computing into supply chain operations has significantly enhanced the capabilities of cyber risk analytics. Organizations can identify and mitigate cyber risks in real-time by processing and analyzing data at the edge of the network. This enables them to take proactive measures to mitigate these risks, thereby enhancing the overall security of their supply chain operations.
5. Graph Analytics
Graph analytics, a part of Graph Data Science, has emerged as a vital technology in managing and analyzing supply chain cyber risks. It employs graph theory to model, analyze, and visualize relationships and interconnections in data.
Graph analytics can quickly uncover complex relationships within supply chain cyber risk data by leveraging data to identify potential disruptions or vulnerabilities.
Graph analytics has seen rapid adoption across various industries due to its ability to manage large volumes of data and provide valuable insights. Gartner predicts a significant increase in the use of graph technologies for data and analytics innovations by 2025.
Procurement firm Scoutbee used graphs analytics to identify patterns in supply chain data, create visualizations of supplier interdependencies, and cut supplier discovery time by 75%.
Graph Analytics and AI in Cyber Risk Analytics
The integration of graph analytics with AI and machine learning is becoming more popular in enhancing cyber risk analysis. This combination leads to more precise predictions and identification of patterns that indicate cyber threats or anomalies. Graph analytics can identify complex patterns within data through deep-link pattern discovery, thereby assisting in the prediction and prevention of cyber threats within the supply chain.
Digital Twins and Graph Analytics
Graph analytics-powered digital twins offer a comprehensive view of the supply chain, identifying areas of risk and mapping out the network. This technology enables organizations to carry out what-if analyses, helping to prevent potential disruptions or risks.
By offering a detailed perspective of the supply chain, graph analytics helps in both tactical and strategic decision-making processes, allowing organizations to respond to changes and potential risks proactively.
6. Blockchain
Blockchain is an emerging technology that could reduce the cybersecurity risks intrinsic to supply chains. It creates an auditable, immutable, unchangeable history of transactions that can be tied to a verifiable identity. It enables better coordination during transactions and reduces risks associated with data leakage and breaches. The decentralized structure of blockchain reduces the risk of single points of failure in supply chains, a common target in cyber attacks. This feature of blockchain can significantly contribute to managing supply chain risks.
Cyber Risk Management in Supply Chains
- Vendor Risk Management: With the complex web of vendors and third parties involved in supply chains, blockchain can help manage and authenticate the identities and activities of all parties, thereby mitigating the risk of cyber threats originating from third parties.
- Traceability and Transparency: Blockchain enhances the traceability of transactions and activities across the supply chain, enabling better monitoring and detection of unusual or suspicious activities that could signify cyber threats.
- Smart Contracts for Security Protocols: Utilizing smart contracts in blockchain can automatically enforce security protocols and compliance standards among all parties in the supply chain.
- Incident Response and Recovery: Blockchain can aid in quicker and more efficient incident response and recovery by providing a clear, unalterable record of all transactions and interactions within the supply chain.
Innovative Supply Chain Cyber Risk Analytics Strategies and Applications
Quantitative Cyber Supply Chain Risk Analysis
Quantitative analysis stands out for its data-driven approach. Unlike qualitative methods that rely on subjective assessments, this technique uses numerical data to evaluate risk levels precisely. It offers an objective lens to view the cybersecurity landscape, quantifying threats to deliver a clear and actionable risk profile.
The quantitative analysis integrates seamlessly with other cutting-edge technologies like AI, blockchain, and predictive analytics. While AI uncovers patterns and predicts potential vulnerabilities, quantitative analysis assigns specific risk values, bringing clarity to these predictions. Similarly, in blockchain-secured supply chains, this analysis quantitatively assesses the impact of potential breaches, adding a new dimension to blockchain’s inherent security.
While promising, quantitative analysis is not without challenges. The accuracy of this approach heavily depends on the quality and completeness of the data. Inconsistent or incomplete data can lead to inaccurate risk assessments. Furthermore, as cyber threats evolve rapidly, keeping the risk model updated with the latest threat intelligence is crucial for its effectiveness.
Real-Time Monitoring and Anomaly Detection
Real-time monitoring and anomaly detection represent a proactive stance in cybersecurity. Leveraging AI and the Industrial Internet of Things (IIoT), this strategy involves continuous surveillance of supply chain operations to identify unusual activities that could signal a cybersecurity threat. AI algorithms learn normal operational patterns and can quickly flag deviations, while IIoT devices provide a stream of real-time data from various points in the supply chain. This combination allows for the immediate detection of potential threats, significantly reducing response times and mitigating potential damage.
Advanced Encryption Techniques
Advanced encryption techniques are critical in safeguarding data across supply chains. As information travels through various nodes in a supply chain, it becomes vulnerable to interception or tampering. Encryption converts this data into a secure format that can only be accessed by authorized parties with the decryption key. This method is essential not only for protecting sensitive information but also for ensuring the integrity of data, as any unauthorized changes can be easily detected.
Behavioral Analytics
Behavioral analytics in supply chain cyber risk management involves using machine learning algorithms to analyze behavior patterns within the supply chain’s digital infrastructure. This method identifies deviations from normal behavior, which can indicate a security breach, such as an insider threat or a compromised account. By understanding typical user behaviors, the system can flag unusual activities, providing early warnings of potential security incidents.
Supply Chain Segmentation
Supply chain segmentation is a strategic approach that involves dividing the supply chain into distinct, manageable segments, each with its own security protocols and controls. This method limits the spread of cyber threats within the supply chain. If a breach occurs in one segment, it can be contained, preventing it from affecting the entire network. Segmentation also allows for more targeted and effective risk management practices tailored to the specific needs and vulnerabilities of each segment.
Cyber Threat Intelligence Platforms
Cyber threat intelligence platforms are dedicated systems that collect, analyze, and disseminate information about current and emerging cyber threats. These platforms utilize advanced AI algorithms to process vast amounts of data from various sources, providing businesses with actionable intelligence. By staying informed about potential cyber threats, companies can proactively adjust their cybersecurity strategies, enhancing their preparedness and response to these threats.
Cloud Security Posture Management (CSPM)
Cloud Security Posture Management (CSPM) is a critical component in managing cyber risks in cloud-based supply chain operations. CSPM tools continuously monitor and manage the security posture of cloud environments, ensuring configurations are in line with best practices and compliance requirements. As supply chains increasingly rely on cloud services for flexibility and efficiency, CSPM ensures these services are not a weak point in the cybersecurity chain, safeguarding data and applications hosted in the cloud.
Bottom Line
The integration of technologies like AI, blockchain, and predictive analytics represents the future of supply chain cyber risk analytics. However, challenges such as technological complexity, evolving cyber threats, and data privacy concerns remain significant. Looking ahead, innovations in AI and machine learning promise more proactive risk management, while emerging technologies like quantum computing hold potential for both challenges and solutions in cybersecurity. The key lies in adapting an integrated approach that combines these technologies with strategic planning to build resilient, secure supply chains.
Innovative Supply Chain Cyber Risk Analytics: Empower Supply Chain Resilience